Discovery of topological constraints on spatial object classes using a refined topological model
Keywords:
topology, classification, OpenStreetMap, data mining, machine learning, frequent itemset mining, qualitative relationsAbstract
In a typical data collection process, a surveyed spatial object is annotated upon creation, and is classified based on its attributes. This annotation can also be guided by textual definitions of objects. However, interpretations of such definitions may differ among people, and thus result in subjective and inconsistent classification of objects. This problem becomes even more pronounced if the cultural and linguistic differences are considered. As a solution, this paper investigates the role of topology as the defining characteristic of a class of spatial objects. We propose a data mining approach based on frequent itemset mining to learn patterns in topological relations between objects of a given class and other spatial objects. In order to capture topological relations between more than two (linear) objects, this paper further proposes a refinement of the 9-intersection model for topological relations of line geometries. The discovered topological relations form topological constraints of an object class that can be used for spatial object classification. A case study has been carried out on bridges in the OpenStreetMap dataset for the state of Victoria, Australia. The results show that the proposed approach can successfully learn topological constraints for the class bridge, and that the proposed refined topological model for line geometries outperforms the 9-intersection model in this task.
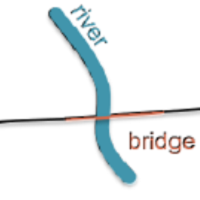
Downloads
Published
Issue
Section
License
Copyright (c) 2019 Ivan Majic, Elham Naghizade, Stephan Winter, Martin Tomko

This work is licensed under a Creative Commons Attribution 4.0 International License.
Articles in JOSIS are licensed under a Creative Commons Attribution 3.0 License.