Inferring routing preferences from user-generated trajectories using a compression criterion
DOI:
https://doi.org/10.5311/JOSIS.2023.26.256Keywords:
trajectory mining, bicriteria optimization, compression, routing preferences, volunteered geographic informationAbstract
The optimal path between two vertices in a graph depends on the optimization objective, which is often defined as a weighted sum of multiple criteria. When integrating two criteria, their relative importance is expressed with a balance factor α. We present a new approach for inferring α from trajectories. The core of our approach is a compression algorithm that requires a graph G representing a transportation network, two edge costs modeling routing criteria, and a path P in G representing the trajectory. It yields a minimum subsequence S of the sequence of vertices of P and a balance factor α, such that the path P can be fully reconstructed from S, G, its edge costs, and α. By minimizing the size of S over α, we learn the balance factor that corresponds best to the user's routing preferences. In an evaluation with crowd-sourced cycling trajectories, we weigh the usage of official signposted cycle routes against other routes. More than 50% of the trajectories can be segmented into five optimal sub-paths or less. Almost 40% of the trajectories indicate that the cyclist is willing to take a detour of 50% over the geodesic shortest path to use an official cycle path.
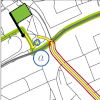
Downloads
Published
Issue
Section
License
Copyright (c) 2023 Axel Forsch, Johannes Oehrlein, Benjamin Niedermann, Jan-Henrik Haunert

This work is licensed under a Creative Commons Attribution 3.0 Unported License.
Articles in JOSIS are licensed under a Creative Commons Attribution 3.0 License.