Service quality monitoring in confined spaces through mining Twitter data
Keywords:
service quality, public transport, event detection, fine-tuned BERT, aspect-based sentiment analysis, statistical analysisAbstract
Promoting public transport depends on adapting effective tools for concurrent monitoring of perceived service quality. Social media feeds, in general, provide an opportunity to ubiquitously look for service quality events, but when applied to confined geographic area such as a transport node, the sparsity of concurrent social media data leads to two major challenges. Both the limited number of social media messages--leading to biased machine-learning--and the capturing of bursty events in the study period considerably reduce the effectiveness of general event detection methods. In contrast to previous work and to face these challenges, this paper presents a hybrid solution based on a novel fine-tuned BERT language model and aspect-based sentiment analysis. BERT enables extracting aspects from a limited context, where traditional methods such as topic modeling and word embedding fail. Moreover, leveraging aspect-based sentiment analysis improves the sensitivity of event detection. Finally, the efficacy of event detection is further improved by proposing a statistical approach to combine frequency-based and sentiment-based solutions. Experiments on a real-world case study demonstrate that the proposed solution improves the effectiveness of event detection compared to state-of-the-art approaches.
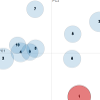
Downloads
Published
Issue
Section
License
Copyright (c) 2020 Mohammad Masoud Rahimi, Elham Naghizade, Mark Stevenson, Stephan Winter

This work is licensed under a Creative Commons Attribution 4.0 International License.
Articles in JOSIS are licensed under a Creative Commons Attribution 3.0 License.