A hidden Markov model for matching spatial networks
Keywords:
spatial networks, data matching, data integration, topology, hidden Markov model, HMMAbstract
Datasets of the same geographic space at different scales and temporalities are increasingly abundant, paving the way for new scientific research. These datasets require data integration, which implies linking homologous entities in a process called data matching that remains a challenging task, despite a quite substantial literature, because of data imperfections and heterogeneities. In this paper, we present an approach for matching spatial networks based on a hidden Markov model (HMM) that takes full benefit of the underlying topology of networks. The approach is assessed using four heterogeneous datasets (streets, roads, railway, and hydrographic networks), showing that the HMM algorithm is robust in regards to data heterogeneities and imperfections (geometric discrepancies and differences in level of details) and adaptable to match any type of spatial networks. It also has the advantage of requiring no mandatory parameters, as proven by a sensitivity exploration, except a distance threshold that filters potential matching candidates in order to speed-up the process. Finally, a comparison with a commonly cited approach highlights good matching accuracy and completeness.
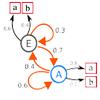
Downloads
Published
Issue
Section
License
Copyright (c) 2019 Benoit Costes, Julien Perret

This work is licensed under a Creative Commons Attribution 4.0 International License.
Articles in JOSIS are licensed under a Creative Commons Attribution 3.0 License.