Evaluating existing manually constructed natural landscape classification with a machine learning-based approach
Keywords:
variable importance, post-classification, validation, geographic information systems, SloveniaAbstract
Some landscape classifications officially determine financial obligations; thus, they must be objective and precise. We presume it is possible to quantitatively evaluate existing manually constructed classifications and correct them if necessary. One option for achieving this goal is a machine learning method. With (re)modeling of the landscape classification and an explanation of its structure, we can add quantitative proof to its original (qualitative) description. The main objectives of the paper are to evaluate the consistency of the existing manually constructed natural landscape classification with a machine learning-based approach and to test the newly developed general black-box explanation method in order to explain variable importance for the differentiation between natural landscape types. The approach consists of training a model of the existing classification and a general method for explaining variable importance. As an example, we evaluated the existing natural landscape classification of Slovenia from 1998, which is still officially used in the agricultural taxation process. Our results showed that the modeled classification confirms the original with a high rate of agreement--94%. The complementary map of classification uncertainty (entropy) gave us more information on the areas where the classification should be checked, and the analysis of the variable importance provided insight into the differentiation between types. Although the selection of the exclusively climatic variables seemed unusual at first, we were able to understand "the computer's logic" and support geographical explanations for the model. We conclude that the approach can enhance the explanation and evaluation of natural landscape classifications and can be transparently transferred to other areas.
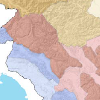
Downloads
Published
Issue
Section
License
Copyright (c) 2019 Rok Ciglič, Erik Štrumbelj, Rok Češnovar, Mauro Hrvatin, Drago Perko

This work is licensed under a Creative Commons Attribution 4.0 International License.
Articles in JOSIS are licensed under a Creative Commons Attribution 3.0 License.